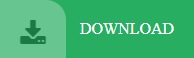
investigated neurologists’ visual attention during CT screening of brain cerebrovascular diseases. They found clear steps which radiologists normally follow sequentially and systematically during diagnostic image scanning. investigated the glioma diagnosis process using multiple MRI sequences by analysing temporal fixation organisation, number of returns to a location, and saccade length. However, there is significantly limited eye-tracking research related to the interpretation of brain images. The bulk of the research on radiologists’ visual scan patterns has focused on breast and chest image interpretation. They used fractal analysis to quantify visual search and receiver operator characteristic (ROC) for diagnostic decisions analysis to differentiate the participant groups and found that gaze complexity is dependent on the image reader characteristics and properties of images. investigated the relationship between image readers’ characteristics and the images by analysing gaze complexity of readers in mammographic mass detection. The difference between groups was greater for diagnostic accuracy compared with visual search metrics. studied the progression of radiologic interpretation skill in chest radiographs by comparing diagnostic accuracy and visual scanpaths among four clinical groups.
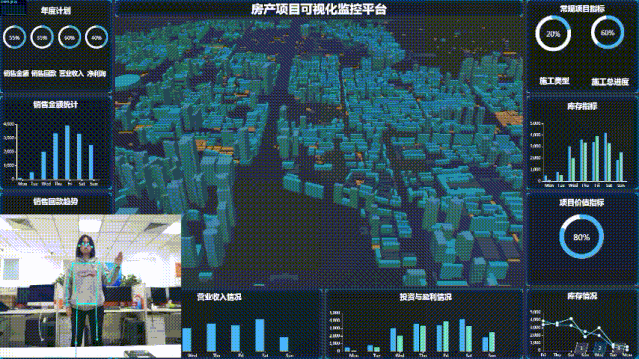
They found that consultant radiologists demonstrated superior pattern recognition skills compared with naïves and trainees. performed an eye tracking study on radiologists with expertise in skeletal imaging who varied in level of experience. They reported that expert radiologists develop global processes for image reading, which guides them to find abnormalities more efficiently than less experienced counterparts. proposed the concept of global perceptual processing during radiologic image reading. The author investigated dwell time between the two groups and found that non-experts spent more time on image searching with larger spatial coverage. Krupinski examined the radiologists’ experience on gaze behaviour by comparing scanpaths between three expert mammographers and three radiology residents. Most studies related to eye-tracking were performed by comparing the gaze behavior of experts and novices. examined the eye-gaze patterns of radiologists when searching for lung cancers in chest radiographs and identified three types of errors that occur when searching a medical image for pathology: (1) visual search errors, where they never fixate on the abnormality (30%) (2) recognition errors, where the abnormality is fixated but may be camouflaged in the image and therefore no meaning is assigned to the area (25%) and (3) decision errors, where the abnormality is fixated but actively dismissed as an abnormality (45%). A large amount of research related to radiologists’ eye movements has been carried out using eye-tracking data to find the relationship between expert radiologists’ search patterns and diagnostic expertise (e.g., recognition of abnormal findings in the radiological images). Therefore, there has been considerable research focused on medical diagnostic errors in recent years. However, diagnosis can be susceptible to human errors causing missed recognition of pathologies. Radiologists screen medical images to detect pathologies. Radiological imaging is the diagnostic workhorse in medicine. The funders had no role in study design, data collection and analysis, decision to publish, or preparation of the manuscript.Ĭompeting interests: The authors have declared that no competing interests exist.
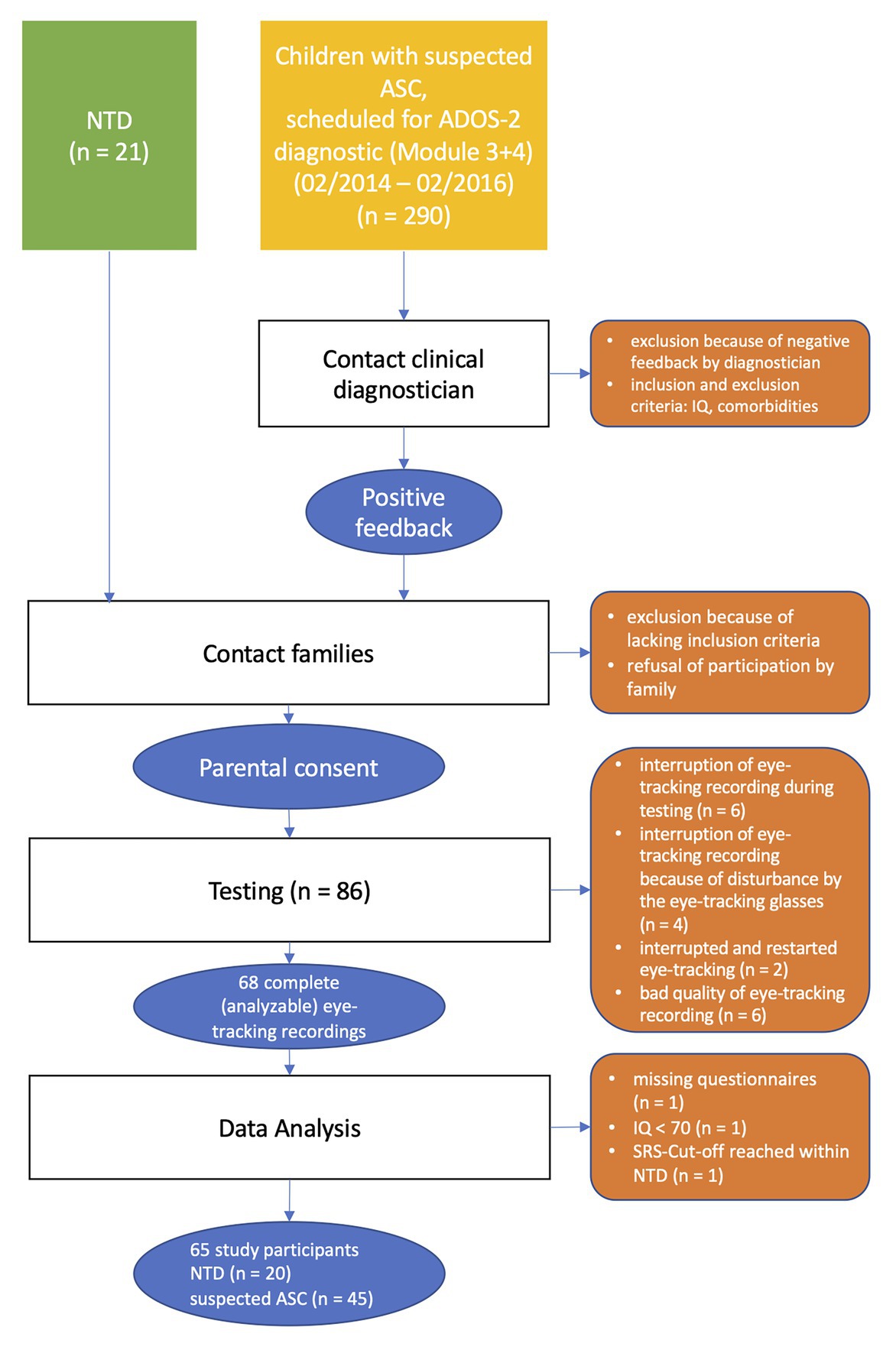
Antonio Di Ieva in 2019 (080619) and by an Australian Research Council (ARC) Future Fellowship granted to Prof. įunding: This work was funded by the Centre for Elite Performance, Expertise & Training (Macquarie University, Sydney) Seeding Grant awarded to Dr Patrick Nalepka, Dr Ann Carrigan, & Prof.
#Eye tracking data analysis code
This is an open access article distributed under the terms of the Creative Commons Attribution License, which permits unrestricted use, distribution, and reproduction in any medium, provided the original author and source are credited.ĭata Availability: All relevant data files and code are available from the following Github public repository link. Received: JAccepted: NovemPublished: December 2, 2021Ĭopyright: © 2021 Suman et al. (2021) Spatial and time domain analysis of eye-tracking data during screening of brain magnetic resonance images. Citation: Suman AA, Russo C, Carrigan A, Nalepka P, Liquet-Weiland B, Newport RA, et al.
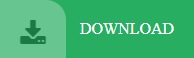